Natural Language Processing Natural Language refers to the regular human language used for communication, and it is the primary mode of human communication. It is also considered the quintessential paradigm of what it means to be intelligent. Effective communication in human language has been deemed as the test of "true" intelligence that any artificial system must pass to be considered intelligent. The famous Turing test was based on this premise, postulating that an AI agent would be considered intelligent if it could converse with a human counterpart in such a manner that the human could not tell if they were conversing with a machine or another human being. One of the main challenges for computers to effectively process language was to turn it into computer-understandable form. This challenge has been addressed by converting written text, primarily in digital form, into some kind of numerical representation. The most commonly used method involves splitting text into small "chunks" called tokens and assigning a string of numbers to each token. Once this "tokenization" has been accomplished, the full range of machine learning tools becomes available to convert the problem of text representation into another machine learning problem. Generative Artificial Intelligence Traditionally, the two most common ways of dividing machine learning problems have been into the so-called supervised and unsupervised problems. Supervised problems are those where we are trying to predict something from the data based on what we already know about each data point. This knowledge is termed “data labels”, and the goal of the supervised learning mechanism is to predict labels for the new data points based on the labels that have been provided for the old data points. For unsupervised learning, however, no such set of data points exists. Unsupervised learning tries to get some kind of insight from the data itself, with any additional information than the structure of the dataset. Over the years yet another paradigm emerged: self-supervised learning. This is sort of a middle ground between supervised and unsupervised learning. The idea behind self- supervised learning is to use select aspects of the dataset as labels for other parts of the dataset. In the ultimate case of this approach, each aspect of the dataset is used as the label for all the other aspects of the dataset. For instance, we can learn the next word in some text based on several other words in the text, or we can try to predict a portion of an image based on all the other aspects of the image, or what an image should look like based on its textual description. Once we build a self-supervised model, we can start using this model to generate new text, images, songs, and so on, based on a small initial “prompt” that we provide. This is the essence of Generative AI. Generative AI has been in development for years, but only in the recent few months has it really captured the world’s attention thanks to the increasing sophistication of the latest generation of models like ChatGPT, Stable Diffusion, and so on. The reason that it took so long to get to this point is that for these Gen AI: Revolutionizing the Way Enterprises Work 10
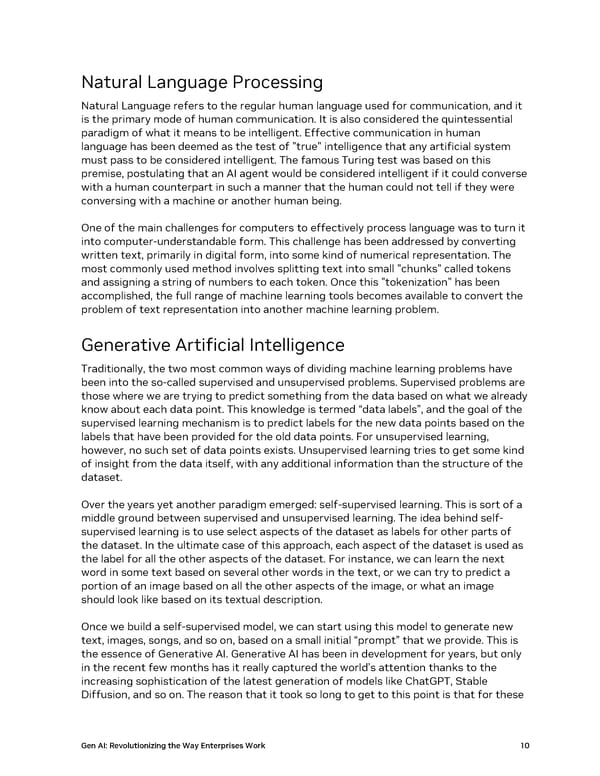