coordination of this signal processing between many, many neurons that characterizes all of the neural processes. Our brains are particularly large agglomerations of neurons, and the complexity of our thoughts is the direct consequence of this extremely large size. Natural neurons are extremely complex cells, and proper simulation of even a single neuron is exceedingly complex. Nonetheless, the early machine learning researchers, in a tried and tested tradition of mathematical modeling of physical phenomena, have been able to reduce this immense complexity to a rather simple set of mathematical functions. Early research with individual neurons hit a snag when it was shown that there were limitations to the kinds of predictive functions it was possible to model with a single neuron. Eventually, it was shown that these setbacks could be overcome when a large combination of such functions was used. Nonetheless, as the number of individual artificial neurons grew, it became increasingly difficult to “train” them properly, and the research on ANNs hit another snag. It is important to understand that even though the inspiration for ANNs came from the natural neural tissue, the analogy should not be taken too literally or too far. Modern ANNs have moved significantly away from the simple original functional representations, and in terms of how they are designed and trained; there is very little remaining similarity with the natural neural networks, except perhaps on a very high level. Deep Learning As mentioned above, training and the expansion of the simple neural networks beyond a few layers of neurons (the so-called “Multi-layer perceptions, MLPs) proved to be a challenge. The need for much more complex neural networks arose as the complexity of data that they needed to be trained on increased. Neural Networks that needed to Artificial Intelligence Thinking machines have been conceived of in some form for almost as long as humans tinkered with machines of any kind, but the modern approach to this field dates from the early 1950s when the term Artificial Intelligence (AI) was conceived. Over the decades there have been many different approaches to the problem of solving intelligence with artificial means, including advanced logic, probabilistic approaches, modeling with knowledge graphs, etc. However, over the years modeling thinking and cognition with Machine Learning and Deep Learning in particular became the prevailing paradigm. AI is still a broad field, and there are still many issues with applying ML across the board (there are many amusing examples where ML-based AI gets even some simple numerical calculations completely wrong), but the advances in Deep Learning over the past 5-10 years have solidified it as the definitive way of tackling the challenge of AI. Gen AI: Revolutionizing the Way Enterprises Work 9
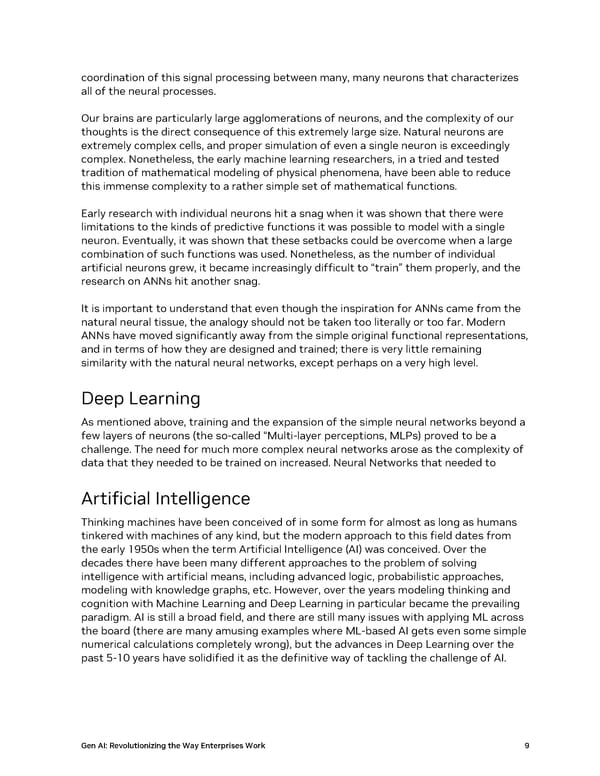